How Netflix & Amazon Personalize Choices using AI
Explore how AI powers recommendation systems on Netflix and Amazon, delivering personalized suggestions to enhance your viewing and shopping experience.
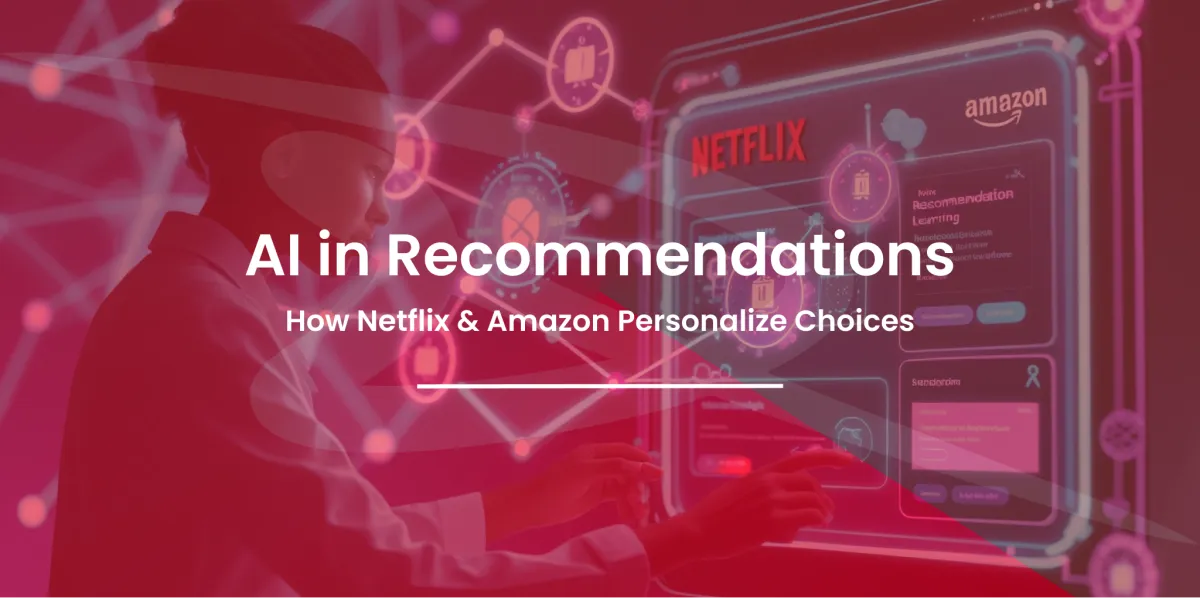
Introduction
In today’s digital world, AI-driven recommendation systems play a crucial role in enhancing user experience by delivering personalized recommendations. Platforms like Netflix and Amazon leverage artificial intelligence to analyze vast amounts of data and predict user preferences, ensuring that consumers engage more with content and products tailored to their interests. This not only boosts customer satisfaction but also significantly impacts business growth by increasing retention rates and sales.
Recommendation systems have revolutionized the way users interact with online platforms. Whether it’s suggesting the next binge-worthy series on Netflix or recommending the perfect product on Amazon, AI algorithms work behind the scenes to provide highly relevant suggestions. These systems utilize machine learning techniques, collaborative filtering, and deep learning models to continuously refine their accuracy. By analyzing user behavior, browsing history, and purchasing patterns, AI ensures that recommendations align closely with individual tastes.
Netflix’s recommendation engine is a prime example of AI-driven personalization. By tracking watch history, ratings, and viewing habits, the platform curates a list of shows and movies uniquely suited to each viewer. Similarly, Amazon employs AI to deliver product recommendations, suggesting items based on past purchases, search queries, and user interactions. This approach not only simplifies the shopping experience but also increases conversion rates for sellers.
The core of AI-powered recommendation systems lies in their ability to adapt and learn from user interactions. Unlike traditional rule-based methods, modern AI-driven recommendations evolve over time, becoming more refined as they gather more data. However, while these technologies enhance user experience and drive engagement, they also raise concerns about data privacy and algorithmic biases.
As AI continues to advance, recommendation systems will become even more precise, transforming the way consumers discover content and products. Understanding how AI powers recommendation engines like Netflix and Amazon provides valuable insights into the future of digital personalization and e-commerce.
Understanding Recommendation Systems
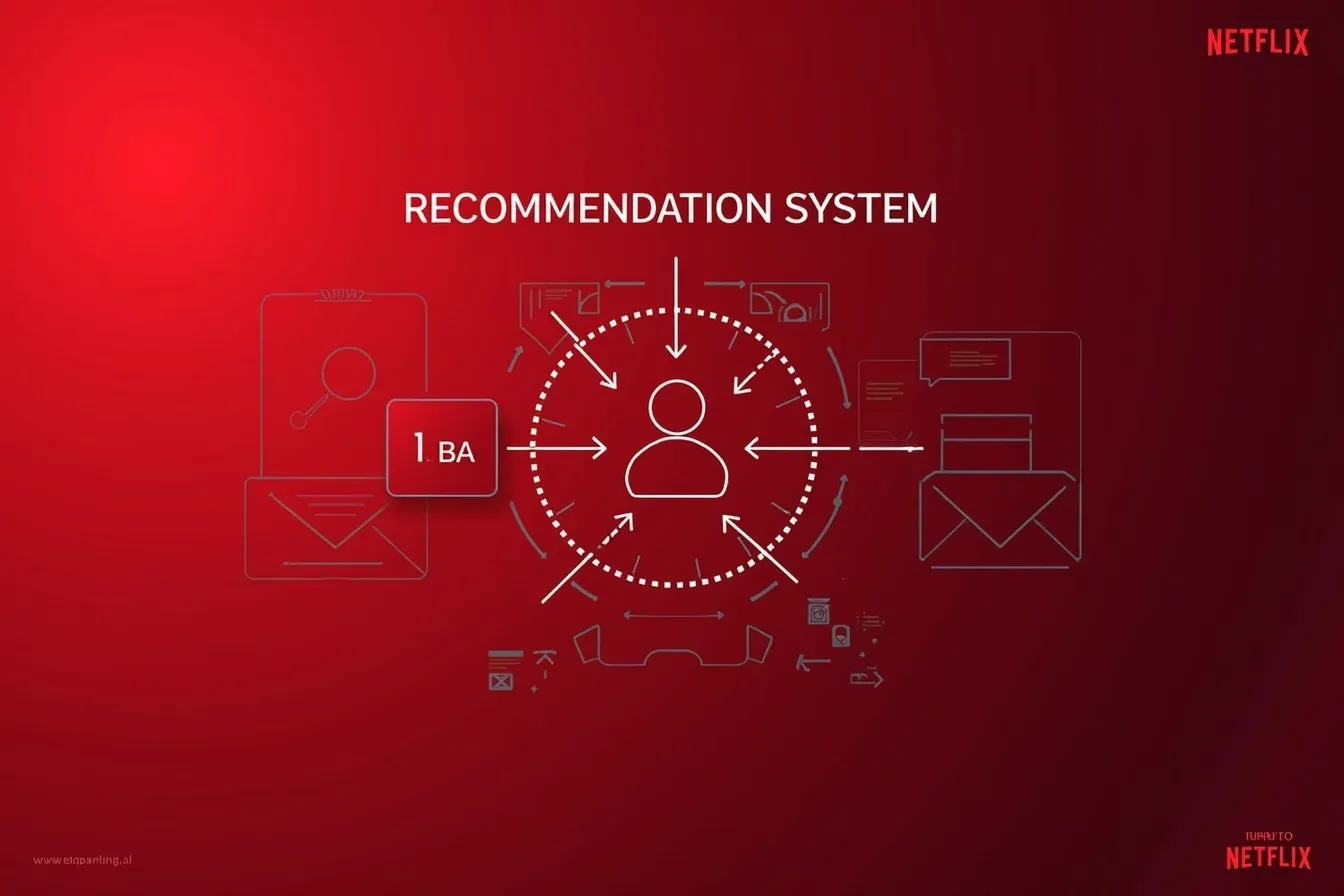
What Are Recommendation Systems?
Recommendation systems are AI-driven technologies designed to suggest relevant content, products, or services to users based on their preferences and behaviors. These systems play a vital role in enhancing user experience by offering personalized recommendations that align with individual tastes and interests. They are widely used across industries, including e-commerce, streaming services, and online marketplaces.
How Do Recommendation Systems Work?
Recommendation systems function by analyzing large datasets containing user interactions, search history, and purchase patterns. They utilize advanced AI techniques, such as machine learning and deep learning, to identify trends and predict what users might find interesting. The effectiveness of these systems depends on their ability to process data in real time and adapt to changing user behavior.
Types of Recommendation Systems
- Content-Based Filtering – This approach suggests items similar to those a user has previously interacted with. For example, if a Netflix user watches several sci-fi movies, the system will recommend more content within that genre.
- Collaborative Filtering – This method identifies patterns in user behavior and suggests items based on similarities with other users. For example, Amazon recommends products based on what customers with similar interests have purchased.
- Hybrid Recommendation Systems – Combining both content-based and collaborative filtering, hybrid systems deliver more accurate and personalized recommendations. Netflix and Amazon both utilize this approach to enhance their recommendation accuracy.
Importance of Recommendation Systems
The impact of recommendation systems is profound, as they:
- Improve user engagement and satisfaction
- Increase conversion rates and sales
- Help businesses retain customers by offering relevant content and products
- Enhance content discovery, ensuring users find new items they might not have searched for otherwise
As AI continues to evolve, recommendation systems will become even more sophisticated, delivering hyper-personalized experiences that redefine the way consumers interact with digital platforms.
How AI and Machine Learning Enhance Recommendation Systems
Role of AI in Personalization
Artificial Intelligence is the backbone of modern recommendation systems, allowing platforms like Netflix and Amazon to create highly customized user experiences. AI algorithms analyze large datasets to detect patterns, understand user preferences, and deliver content that aligns with individual tastes. This personalization ensures that users are more likely to engage with recommendations, improving retention and satisfaction.
Machine Learning Algorithms in Recommendation Systems
Machine learning plays a critical role in refining recommendation systems. Some key techniques include:
- Supervised Learning – AI models are trained on labeled data, learning from user behaviors to make accurate predictions. For instance, Netflix uses supervised learning to suggest shows based on users’ past viewing history.
- Unsupervised Learning – This approach clusters users with similar behaviors to make recommendations. Amazon groups customers with similar purchase habits to suggest products that may interest them.
- Deep Learning – Advanced neural networks analyze complex patterns in user interactions. These models help platforms refine recommendations over time, improving the relevance of suggestions based on evolving user behavior.
How AI Adapts to User Behavior
One of AI’s key strengths is its ability to adapt to changing user preferences. Unlike traditional recommendation methods that rely on static rules, AI-driven systems continuously learn and evolve. By monitoring user interactions, these systems:
- Adjust recommendations in real time
- Improve accuracy by considering context, such as time of day or seasonality
- Reduce irrelevant suggestions by filtering out content that users skip or dislike
AI’s Impact on Recommendation Accuracy
AI enhances recommendation accuracy by:
- Understanding user intent based on subtle behavioral cues
- Analyzing large-scale data to identify emerging trends
- Optimizing search results and suggestions based on user engagement
By integrating AI and machine learning, recommendation systems become smarter, delivering personalized content that keeps users engaged and satisfied. As these technologies continue to evolve, they will further enhance digital experiences across industries.
AI in Netflix’s Recommendation Engine
Netflix’s recommendation engine is one of the most advanced AI-driven systems, designed to enhance user experience by delivering personalized recommendations based on user preferences. With millions of subscribers worldwide, Netflix relies on machine learning algorithms and behavioral patterns to ensure that each viewer receives content suggestions tailored to their tastes.
How Netflix Uses AI for Recommendations
Netflix’s AI-driven system analyzes viewing history, watch duration, interactions (such as likes, ratings, and skips), and behavioral patterns to predict what users might want to watch next. It employs several AI techniques, including:
- Collaborative Filtering – Identifies similarities between users and suggests content based on shared interests.
- Content-Based Filtering – Recommends shows and movies similar to what a user has previously watched.
- Deep Learning Models – Uses neural networks to refine recommendations by evaluating intricate patterns in user behavior.
Enhancing Content Discovery
Netflix continuously improves its recommendation engine by adapting to real-time data. The platform ensures content discovery by offering categories like “Trending Now,” “Because You Watched,” and “Top Picks for You.” These sections are dynamically curated using AI-driven recommendations to maximize engagement.
By leveraging AI, Netflix not only boosts watch time but also strengthens customer retention, making it a leader in personalized content streaming.
Challenges & Ethical Concerns in AI-Powered Recommendations
AI-powered recommendation systems have transformed how users discover content and products, making platforms like Netflix and Amazon more engaging and efficient. However, these systems also pose significant challenges and ethical concerns, including data privacy, algorithmic bias, transparency, and user autonomy. As AI continues to shape digital experiences, addressing these issues becomes crucial for maintaining trust and fairness in recommendations.
1. Data Privacy and Security
One of the biggest concerns in AI-driven recommendation systems is data privacy. These systems collect vast amounts of user data, including browsing history, viewing preferences, search queries, and purchase behavior. While this data is essential for personalized recommendations, it raises serious questions about how it is stored, shared, and protected.
- Risk of Data Breaches – If not properly secured, sensitive user data can be exposed to cyberattacks, leading to privacy violations.
- User Consent Issues – Many platforms collect data without explicitly informing users how their information is used for recommendations.
To address these challenges, companies must implement robust security protocols, adopt transparent data policies, and give users control over their personal information.
2. Algorithmic Bias and Fairness
AI models learn from historical data, which can sometimes lead to algorithmic bias. If training data contains biases, the recommendation system may reinforce unfair or discriminatory patterns.
- Content Diversity Issues – AI may prioritize mainstream content while neglecting niche genres, leading to a lack of diverse recommendations.
- Demographic Bias – If an algorithm is trained on a limited dataset, it may favor certain user groups while underrepresenting others.
Companies must adopt fair AI training practices, ensure diverse datasets, and conduct regular audits to minimize bias in recommendations.
3. Lack of Transparency
Most AI recommendation engines function as black-box models, meaning users do not fully understand how or why certain recommendations appear. This lack of transparency can create distrust, especially when platforms manipulate recommendations for commercial gain.
- Sponsored Content Concerns – Some platforms mix organic and paid recommendations without clearly differentiating them, misleading users.
- Unclear Decision-Making Process – Users may not know how their data influences suggestions, making it difficult to adjust their preferences.
To improve transparency, platforms should provide explanations for recommendations, such as why a particular movie or product was suggested. User education on AI-driven personalization can also enhance trust.
4. Over-Personalization and Filter Bubbles
AI aims to provide highly personalized recommendations, but excessive personalization can create filter bubbles—where users are repeatedly shown content similar to their past choices, limiting their exposure to new perspectives.
- Echo Chambers – Users may only see information that reinforces their existing preferences, reducing diverse content discovery.
- Reduced Exploration – Over-reliance on AI recommendations can prevent users from discovering unique or unexpected content.
To counter this issue, platforms should introduce serendipity algorithms, which occasionally suggest content outside a user’s typical preferences to encourage exploration.
5. Ethical Use of AI in Recommendations
With the increasing influence of AI-driven recommendations, ethical concerns around manipulation and user autonomy have emerged. Some platforms optimize recommendations for engagement metrics rather than user well-being, leading to concerns such as:
- Addictive Content Consumption – AI may prioritize content that keeps users engaged for longer, potentially leading to excessive screen time.
- Monetization Strategies – Platforms may push recommendations that maximize revenue rather than improve user experience.
To ensure ethical AI usage, companies should balance business objectives with user well-being, prioritizing responsible AI design over pure profit-driven algorithms.
The Future of AI in Recommendation Systems
As artificial intelligence continues to evolve, recommendation systems are poised to become more intelligent, personalized, and efficient. Emerging AI technologies such as deep learning, natural language processing (NLP), and reinforcement learning will redefine how platforms like Netflix, Amazon, and other digital services recommend content and products. The future of AI-powered recommendations will focus on enhancing personalization, improving real-time adaptability, ensuring transparency, and addressing ethical concerns.
1. Hyper-Personalized Recommendations
Future recommendation systems will provide even more precise and personalized suggestions by leveraging advanced deep learning models. These systems will:
- Use context-aware AI to analyze real-time user interactions, mood, and preferences.
- Integrate multimodal learning, combining data from text, images, voice, and video to enhance recommendations.
- Offer emotional AI-powered suggestions, where AI understands emotions through facial recognition, voice tone, or typing patterns.
For example, Netflix may refine its recommendations based on real-time reactions rather than just past viewing history, making suggestions even more dynamic.
2. Real-Time and Adaptive AI Recommendations
Traditional recommendation systems primarily rely on historical data, but the next generation of AI will focus on real-time adaptability. These systems will:
- Instantly adjust recommendations based on live user behavior rather than static data.
- Utilize edge computing to process recommendations on devices rather than relying solely on cloud-based AI models.
- Incorporate reinforcement learning, allowing AI to learn from user feedback in real time to refine its suggestions.
For instance, Amazon’s AI may soon recommend products based on immediate actions, such as hovering over an item, adding it to a wishlist, or scanning reviews.
3. More Transparent and Explainable AI
As concerns about AI bias and black-box algorithms grow, the future of recommendation systems will emphasize explainability and transparency. Future AI systems will:
- Provide clear insights into why specific recommendations are made.
- Allow users to customize AI models, giving them control over how recommendations are generated.
- Implement AI fairness checks to reduce algorithmic bias and ensure ethical recommendations.
Companies will likely introduce user-facing AI dashboards, where people can adjust their recommendation preferences and see how their data influences AI decisions.
4. Ethical AI and Responsible Personalization
The future of AI recommendations will also focus on reducing manipulation and ethical concerns. Platforms will:
- Ensure that AI recommendations prioritize user well-being rather than just engagement.
- Develop responsible AI algorithms that prevent filter bubbles and echo chambers.
- Provide opt-in transparency, allowing users to modify or disable recommendation algorithms.
For example, social media platforms may introduce AI-driven content diversification tools, ensuring users are exposed to a broader range of perspectives and ideas.
5. Voice and Conversational AI in Recommendations
With the rise of voice assistants like Alexa, Google Assistant, and Siri, the future of recommendation systems will integrate voice-based AI interactions. Future AI will:
- Use natural language understanding (NLU) to personalize recommendations through voice commands.
- Enable users to converse with AI to refine suggestions dynamically.
- Provide a seamless omnichannel recommendation experience, synchronizing voice, text, and visual suggestions across devices.
For instance, users could simply ask, "What should I watch tonight?", and AI would provide personalized recommendations based on their past interactions and current mood.
6. Cross-Platform and Multi-Device Recommendations
AI-driven recommendations will become more interconnected across devices and platforms. Instead of recommendations being restricted to a single platform, future AI systems will:
- Create unified AI profiles that sync preferences across multiple services (e.g., Netflix, Spotify, and Amazon).
- Predict user needs across different devices, offering suggestions that seamlessly transition from a smartphone to a smart TV or VR headset.
- Use federated learning, allowing AI to improve recommendations without sharing raw user data, enhancing privacy and security.
For instance, a user watching a cooking show on Netflix may get smart grocery recommendations on Amazon, creating a fully integrated experience.
Conclusion
AI-powered recommendation systems have revolutionized how users discover content, engage with digital platforms, and make purchasing decisions. Platforms like Netflix, Amazon, and other e-commerce giants leverage AI to analyze user behavior, browsing history, and preferences to deliver highly relevant recommendations. By employing machine learning algorithms, deep learning techniques, and collaborative filtering, these systems refine their predictions and enhance customer engagement. Whether it’s personalized movie recommendations on Netflix or intelligent product suggestions on Amazon, AI-driven recommendations have become an integral part of the digital experience.
However, while AI improves recommendation accuracy and boosts user satisfaction, it also brings forth challenges and ethical concerns. Issues such as data privacy, algorithmic bias, and filter bubbles have raised concerns about how AI influences consumer decisions. Over-personalization can limit exposure to diverse content, while the lack of transparency in AI models may lead to trust issues among users. Businesses must strike a balance between effective personalization and responsible AI practices to ensure fairness and accountability.
The Impact and Future of AI-Powered Recommendations
The future of recommendation systems lies in further advancements in deep learning, natural language processing (NLP), and reinforcement learning. These technologies will enable AI to provide even more accurate, real-time, and context-aware recommendations. Additionally, the integration of voice assistants, conversational AI, and multi-platform recommendation engines will create a seamless and personalized user experience across different digital ecosystems.
Despite these advancements, businesses must prioritize ethical AI development by:
- Enhancing transparency in how recommendations are generated.
- Mitigating bias in machine learning models.
- Ensuring data privacy and compliance with regulations.
- Providing user control over personalized recommendations.
- Adopting hybrid recommendation models that combine multiple AI techniques for accuracy and fairness.
Final Thoughts
As AI continues to evolve, recommendation engines will become more sophisticated, intuitive, and user-centric. Businesses that leverage AI-powered recommendations effectively will benefit from higher engagement rates, improved conversion rates, and enhanced customer loyalty. However, responsible AI innovation is key to ensuring that recommendation systems remain transparent, unbiased, and privacy-conscious. The future of AI-driven recommendations will depend on how well companies balance technological advancements with ethical considerations, ultimately shaping a more personalized and fair digital landscape.
Comments ()